As the digital landscape expands, so does the attack surface for potential cybe....
Machine Learning in Cybersecurity: A Proactive Approach
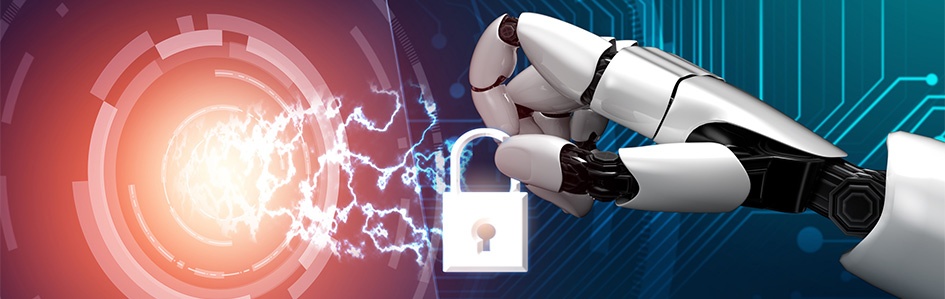
In an era where digital landscapes evolve at an unprecedented pace, the battle between cybersecurity professionals and malicious actors intensifies daily. According to Cybersecurity Ventures, cybercrime is expected to cost the world 10.5 trillion annually by 2025.
The customary reactive strategies, while essential, are no longer sufficient to combat the dynamic and sophisticated nature of cyber threats. Enter machine learning, a game-changing technology that is reshaping the cybersecurity paradigm from a reactive stance to a proactive, preventive one.
What is Machine Learning (ML)?
Machine learning is a branch of artificial intelligence (AI) that empowers computers to learn patterns, make decisions, and improve their performance over time without explicit programming. At its core, machine learning involves the development of algorithms and statistical models that enable machines to analyze data, recognize patterns, and make predictions or decisions autonomously.
It relies on the process of feeding large amounts of data to a computer, allowing it to learn and adapt to complex tasks. The key goal of machine learning is to enable computers to generalize from experience, making them capable of handling new, unseen data and tasks. This technology has found widespread applications in various fields, from image and speech recognition to recommendation systems and predictive analytics.
Introduction to Machine Learning in Cybersecurity
In the dynamic world of cybersecurity, machine learning emerges as a pivotal force reshaping defense strategies. Its role extends beyond conventional measures, offering a proactive approach to threat detection and mitigation. This introduction explores how machine learning analyzes patterns, learns from irregularities, and addresses the shortcomings of traditional cybersecurity methods. Exploring the area of predicting potential threats to continuously addressing them ahead of time highlights the importance of using machine learning to strengthen digital defenses against cyber threats.
As organizations across the globe face the escalating frequency and complexity of cyber-attacks, the integration of machine learning algorithms has emerged as a formidable ally in fortifying digital defenses. The intersection of machine learning and cybersecurity plays a pivotal role in not only detecting and mitigating threats but also in anticipating and neutralizing potential attacks before they unfold.
Proactive Defense Strategies
The standard of cybersecurity is shifting towards a defense strategy, marking a critical departure from reactive approaches. By anticipating and neutralizing potential threats ahead of time, organizations can significantly enhance their resilience. Crucially, the integration of machine learning stands as the cornerstone in this transformative shift, enabling a vigilant stance by leveraging predictive analytics, behavioral analysis, and anomaly detection. Machine learning's capacity to learn and adapt in real-time empowers cybersecurity to evolve from a traditional model to an initiative of defense against evolving digital risks.
Real-World Applications
In the realm of cybersecurity challenges, machine learning emerges as a dynamic ally, showcasing its prowess through numerous real-world applications that redefine the defense landscape.
- Anomaly Detection and Behavioral Analysis
Financial institutions employ machine learning algorithms to analyze transaction patterns, detecting anomalies that signal potential fraudulent activities. Detection of unusual patterns goes beyond rule-based systems, allowing organizations to preemptively block suspicious transactions and thwart financial cybercrime.
- Predictive Threat Intelligence
Security teams utilize machine learning models to analyze vast datasets and predict emerging cyber threats based on historical data and evolving patterns. This anticipatory approach enables organizations to fortify defenses against potential threats before they materialize, ensuring a step ahead in the ongoing battle against cyber risks.
- Adaptive Malware Detection
Antivirus solutions leverage machine learning to dynamically adapt and identify new strains of malware based on behavioral characteristics rather than relying solely on known signatures. Continuous adaptation to evolving malware ensures early detection and containment, mitigating the risks associated with novel and sophisticated threats.
- User Authentication and Access Control
Machine learning algorithms analyze user behavior patterns to create baseline profiles, facilitating the identification of anomalous activities that may indicate unauthorized access. Enhancing traditional access control methods, this approach prevents unauthorized access by identifying deviations from established user behavior norms.
- Incident Response Automation
Machine learning is employed in incident response workflows, automating the analysis of security alerts and prioritizing potential threats based on their severity. Automation accelerates response times, allowing cybersecurity teams to focus on mitigating high-priority threats swiftly, therefore, minimizing potential damage.
- Network Traffic Analysis
Machine learning algorithms scrutinize network traffic patterns, identifying anomalies and potential security breaches. Detection of irregularities ahead of time helps in mitigating the risks associated with advanced persistent threats (APTs) and other network-based attacks.
- Phishing Detection and Email Security
Machine learning models analyze email content and user behavior to identify phishing attempts, malicious links, and suspicious attachments. Identification of phishing threats strengthens email security, preventing users from falling victim to social engineering attacks.
- Proactive Vulnerability Management
Machine learning is applied to identify and prioritize vulnerabilities in software and systems based on historical data and emerging threat trends. Organizations can address vulnerabilities before they are exploited, reducing the attack surface and enhancing overall cybersecurity resilience.
As organizations increasingly adopt these applications, the relationship between human expertise and machine learning algorithms becomes pivotal in achieving a proactive approach to cybersecurity.
Machine Learning and Ethical Considerations
Embarking on the integration of machine learning in cybersecurity represents transformative potential, yet not without navigating a terrain of challenges and considerations. Addressing ethical concerns is crucial, requiring a thorough examination of potential biases in algorithms and models. Transparency is essential to address worries about the interpretability of machine learning decisions.
As organizations adopt prepared defenses with machine learning, it is vital to understand these challenges, ensuring a balanced approach that aligns technological abilities with ethical imperatives in strengthening digital landscapes against cyber threats.
Integrating Machine Learning with Existing Security Infrastructure
The seamless integration of machine learning with existing security infrastructure marks a pivotal stride toward a proactive defense. Rather than requiring a complete overhaul, machine learning harmoniously collaborates with established cybersecurity tools and frameworks, augmenting their capabilities and fortifying the digital fortress.
This collaborative integration empowers organizations to leverage the strengths, of both traditional and cutting-edge technologies, creating a collaboration that enhances overall cybersecurity resilience. Machine learning adapts to the existing security protocols, adding an intelligent layer that can proactively identify and mitigate emerging threats.
Machine Learning in Cybersecurity: Future Trends and Innovations
Peering to the future of cybersecurity, the trajectory shaped by machine learning promises a landscape of innovative advancements and transformative trends. Quantum leaps in technology, such as the integration of quantum computing and the refinement of deep learning architectures, hold the potential to redefine the proactive approach in cyber defense.
The convergence of artificial intelligence and cybersecurity is poised to unveil new dimensions, where machine learning not only anticipates threats but proactively adapts to emerging attack vectors. Continuous innovation in anomaly detection, threat intelligence, and behavioral analytics is on the horizon, promising a more dynamic defense against cyber threats. The intersection of machine learning and cybersecurity continuously becomes the frontier of pioneering solutions, shaping the narrative of resilience in the ongoing battle for digital security.
Conclusion
The landscape of cybersecurity is undergoing a profound transformation, with machine learning standing as a formidable force in ushering a proactive era of defense. From predictive analytics and behavioral analysis to adaptive learning, the multifaceted role of machine learning in fortifying digital landscapes against the relentless tide of cyber threats is becoming more evident. Real-world applications demonstrate its capacity to not only detect, but anticipate and neutralize potential risks.
However, challenges and ethical considerations remain, demanding a balanced integration of technological prowess with ethical imperatives. As we navigate the dynamic terrain of the evolving threat landscape, the collaborative synergy between machine learning and human expertise emerges as paramount.
How Can PECB Help?
PECB's cybersecurity management training courses deliver practical guidance, equipping individuals with the skills to protect organizations against cyber threats.
PECB’s Cybersecurity Management training courses include:
About the Author
Fëllënza Hoxha is the Magazine Editor and Quality Assurance Specialist at PECB. She is in charge of creating and gathering content for the PECB Insights Magazine, along with ensuring content quality. If you have any questions, please do not hesitate to contact her at: insights@pecb.com.